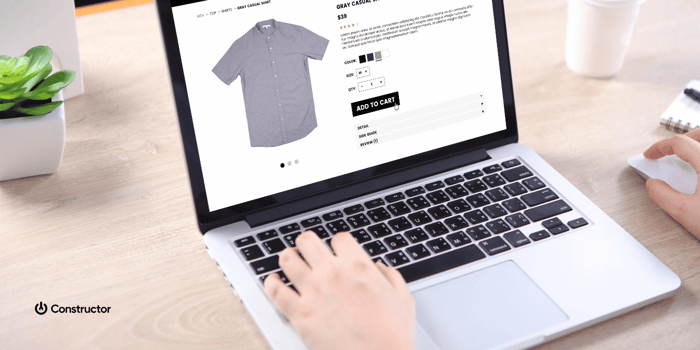
This article was written in collaboration with Valentina Nochka, Product Manager, Ranking, Personalization, and Attribute Enrichment.
Product Badges are becoming increasingly important in ecommerce stores’ visual merchandising strategies. Often, they are designed to take advantage of psychological triggers to drive urgency among shoppers, increase FOMO, and boost sales.
While their effectiveness is widely accepted in the industry, there is little data publicly available that demonstrates how badges influence shopping decisions and the size of their impact on different shopping behaviors.
To help answer these questions and others, Constructor tested badges with one of our largest apparel customers. Read on to learn more about what we found.
What Are Product Badges?
Product Badges are strategic visual markers placed on PLPs to convey key information about products to online shoppers quickly. Badges can be used to highlight specific attributes (such as special features, exclusivity, etc.) and/or communicate an item's popularity (best sellers, selling fast, trending, etc).
Badges mimic certain processes in physical retail environments. For instance, a bestseller is like seeing a product in many people's shopping carts, while trending items are like those displayed on mannequins in a clothing store. In the online space, we often lack this social experience, and one way to simulate it is through badges.
Although the concept sounds simple enough, ecommerce teams often struggle to implement them due to the amount of manual effort typically required. For example:
- Some types of badges (such as Best Sellers) require certain metrics to be calculated, and many existing tools can’t do this automatically.
- Some badges require complicated decision making, such as determining thresholds for when to show products or optimizing how many badges to show per page.
- Badges require a lot of maintenance, especially for large catalogs where new products are constantly being introduced or phased out.
Our Hypothesis and Test Parameters
Last year, Constructor’s ML Ranking team postulated that integrating badges into the ranking and attractiveness layers of our algorithm would both produce better results than traditional methods and make merchandisers’ lives easier by automating the more complicated aspects of configuration.
The core hypothesis was that badges would motivate shoppers to make decisions faster, specifically in situations where there were a large number of options in the results (in other words - reducing the analysis paralysis that sometimes plagues shoppers when too many choices are given). The test parameters were as follows:
- Duration: 1 month
- Results Type: Search and Browse
- Badge Strategies: Best Sellers, Trending, and New Arrivals
- KPIs Monitored (and their definitions):
- Browse clicks = measures the total number of clicks on products on the categories/facets PLPs
- Total clicks per user = measures share of users with click action
- Funnel browse to click = metric tracks how often people browse categories/facets and then click on products. If this number goes up, it means that more people who load the Browse pages are actually clicking on something, indicating that displaying badges makes users more likely to interact with products on PLPs.
What we do differently (and why we predicted results would be positive)
There are a few ways we approached the problem differently that we believed would lead to differentiated results.
First and foremost, our solution handles the calculations required to determine which products can receive a badge, for example, calculating Best Sellers based on sales over a certain period. Specific thresholding can be controlled by the retailer to ensure the number of badges aligns with the strategy and positioning (e.g., some brands prefer a more premium look & feel and therefore fewer badges).
Secondly, because badges are tied to our ranking and attractiveness algorithms, we handle the ordering/positioning of products so that the most attractive products (and those that would benefit the most from a KPI standpoint) receive badges. The badges contribute to the explainability of ranking, highlighting why certain products are ranked higher than others.
Finally, we consider prioritization when applying different types of badges. This also contributes to explainability for shoppers and helps make product positions make sense. As an example, it might raise questions if a brand new item is in Position 1 vs a top-selling product.
The Results
Since the customer we ran this test with received roughly 10x more traffic in browse than search (not uncommon for apparel brands), we were only able to reach statistical significance (statsig) for the following metrics:
- browse clicks: +1.61%
- total clicks per user: +0.9%
- funnel browse to click: +1.5%
We also observed that the number of loaded browse pages (browse_load) decreased, while the number of unique users increased (browse_load_user). We believe these two KPIs are connected, though we can’t say for sure. We didn't check if this decrease was due to the reduction of usage of filters and pagination, but it seems like a signal that we've reduced the cognitive load on users (by decreasing the amount of paginating and hunting they have to do) and helped them navigate their product choices.
What to Conclude
So, what did we ultimately learn?
The metrics show that Badges increased the number of clicks and made shopping easier by reducing the number of pages users had to go through. This supports our idea that badges help reduce decision fatigue, enabling shoppers to make quicker choices.
As ecommerce continues to evolve, leveraging visual cues like badges will be essential for brands aiming to drive urgency and boost sales. The integration of automated badge calculations and prioritization within ranking systems marks a significant step forward for merchandisers, allowing them to focus on strategy rather than manual tasks.