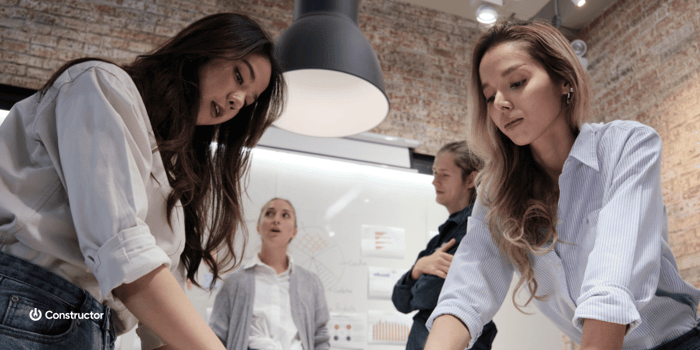
Imagine if predicting hot styles were as simple as consulting a Magic 8 Ball.
Q: “Will fringe make a comeback this spring?”
A: “You may rely on it.” (or: “My sources say no.”)
Then, retailers and their entire operations teams could make decisions accordingly.
But in fashion and apparel, the area of trend forecasting — or predicting popularity to inform upcoming clothing collections — is notoriously tricky, combining both art and science.
The discipline used to be the focus of solely an elite few: fashion experts and forecasters who attended New York and Paris Fashion Weeks and other runway shows, factoring in pop culture when making style predictions.
Editors at esteemed fashion magazines also played a role in shaping and influencing must-have styles. Think of this scene from The Devil Wears Prada when fashion ingenue Andy Sachs (Anne Hathaway) notes incredulously, “So because she [Runway Editor Miranda Priestley] pursed her lips, he’s going to change his entire collection?” Runway’s Art Director Nigel answers: “You still don’t get it, do you? Her opinion is the only one that matters.”
It’s A Whole New (Trendsetting) World
Nowadays, the universe of trendsetters has significantly expanded as celebrities, fashion bloggers, Instagramers, TikTok influencers, and more help dictate what’s popular.
What’s hot and what’s not changes at a breakneck pace, often moving at the speed of social media since the latest trends are just a click away. Eagle-eyed shoppers ID Taylor Swift’s latest Chiefs merch or the Duchess of Sussex’s new knotted earrings, only for those items to sell out within hours.
With fashion trends sometimes fickle and fleeting — and also shaped by emotion, social factors, and prevailing pop culture (think: Barbiecore aesthetic) — forecasting is anything but an easy endeavor.
Though, it’s something fashion and apparel retailers need to get right. Being late to the game or off by a shade (with a spring clothing line in orchid, when pastel violet is what’ll be “in”) can have a meaningful impact on the bottom line.
“A big part of success when working in this industry is agility: paying attention to newness and not ignoring it,” Jeffrey Roy, editorial fashion specialist at a prominent luxury apparel retailer, told Constructor. “You have to be open to ideas that are emerging and never totally know how they’re going to hit.”
Why a manual-only forecasting approach won’t work
Given ever-shifting, interconnected, and sometimes hard-to-predict fashion influences, can retailers take a manual-only approach to forecasting?
“Outlook not so good,” your Magic 8 Ball might answer.
Such an approach is not only time-consuming, but severely limited in its efficacy. It’s prone to error, delayed in reaction time, and even susceptible to the forecaster’s own predilections.
Tapping into Artificial Intelligence
As advanced AI continues to bring efficiencies to the business landscape, the area of trend forecasting is reaping benefits, too.
AI models are standardizing and improving fashion trend forecasting by instantly digesting vast amounts of historical and real-time data, uncovering patterns, and flagging what’ll be noteworthy trends.
In particular, AI can process data such as fashion show images, social media posts, review content, online and in-store sales data, search engine queries, and additional behavioral data to make predictions faster and with greater accuracy than humans.
There are many benefits of improved forecasting with AI, including:
- Delivering a better customer experience, with retail inventory aligned to items customers want to buy.
- Increasing sales, thanks to more on-the-mark items reaching the right consumers.
- Contributing to a much more sustainable fashion landscape, with fewer items getting produced, discarded, and ultimately relegated to landfills. According to Fibre2Fashion, “the fashion industry contributes more to annual global carbon emissions than all international flights and maritime shipping combined, accounting for over 10 percent. Moreover, the greenhouse gas emissions associated with the fashion sector are expected to surge by more than 50 percent by 2030.”
What Are the Best Ways to Use AI for Trend Forecasting?
AI can be applied to improve and accelerate trend forecasting in a number of ways. We’ll explore some common use cases below.
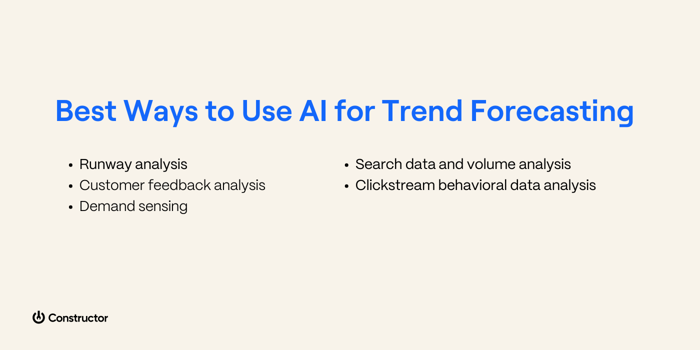
Runway analysis
AI models today can look at how runway styles will permeate and influence luxury and mass market styles. They scan huge collections of images and infer information about popular patterns, cuts, color palettes, and so on.
In addition, search engines such as Tagwalk blend proprietary AI with human tagging to highlight trends so users can search through fashion shows, designers, accessories, and trends by keyword.
Social analysis
AI-based social analysis is a key component in forecasting as social media greatly influences and intersects with the fashion world. Social media grants influencers a platform with broad visibility, enabling fashion brands to interact with their customers while putting trend information at shoppers’ fingertips.
Key players in this space include:
- London-based consultancy WGSN, which uses proprietary AI models that factor in social media and social listening (among other data) in trend forecasting.
- Paris-headquartered Heuritech, offering “AI-powered insights to back fashion intuitions.” Every day, the company analyzes millions of images posted to social media, applying virtual recognition technology to quantify and predict what people wear by market.
- T-Fashion, which uses AI to analyze social data, influencer data, and data points from target audiences to “unveil the future of fashion trends.”
- Trendalytics, interpreting Google Trends information, social media, and ecommerce market data to predict emerging trends (as well as those trends on the road to passé).
Free tools also help surface social trends and highlight what’s going viral. For example, fashion and apparel brands and forecasters can teach TikTok’s algorithm to show them trends related to their market to help stay on top of what’s new.
Customer feedback analysis
Customer feedback analysis involves using AI — specifically natural language processing (NLP) — to extract trends, sentiment, and insights from customer feedback that’s collected in various forms. They include product reviews, online surveys, chatbot interactions, social media posts, etc. Various products address this, such as Akkio, BazaarVoice, and Yotpo.
Brands can also consider feeding data to ChatGPT and similar Generative AI (GenAI) models to rapidly perform sentiment analysis and detect actionable trends from their customer feedback.
Armed with these insights, fashion and apparel brands can then make informed decisions about their offerings and styles.
Demand sensing
While it’s very important to know what’ll be trending seasons in advance, fashion retailers and supply chain planners also need to be able to get a sense for near-term, future demand. What will happen days (or even hours) from now?
Using current demand data in combination with AI, demand sensing solutions can help planners make better short-term decisions and react with confidence. This forecasting method differs from demand planning, which develops strategic forecasts for longer-term horizons (e.g., months and even years out).
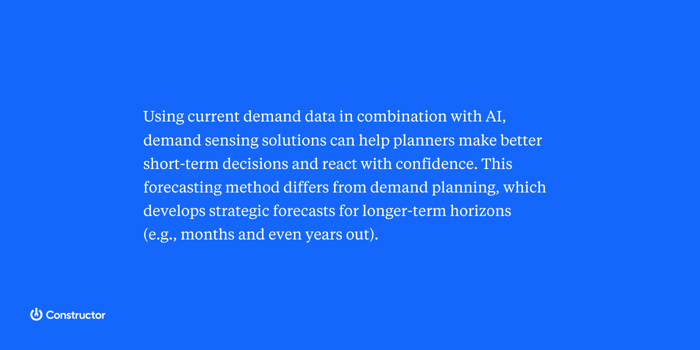
Popular, AI-based demand sensing solutions include Logility, Stylumia, and Woven Insights.
Search data and volume analysis
Shoppers’ search data can also help brands capitalize on trends and make important short- and long-term decisions related to inventory planning, promotions, campaigns, product tagging, and more. The first clue to shoppers’ interests is often the first step in their journey.
Tools like Google Search Console or Semrush, which are incorporating more AI features, will show the search terms or keywords that may have brought a visitor to a retailer’s site in the first place.
And once shoppers land on that site, it’s important for fashion brands to know: what are they doing there? What search terms are they using? Which ones are trending? Which terms are yielding tangible results? Which ones aren’t?
Leading AI-based product discovery solutions provide a user-friendly dashboard with real-time insights. Leveraging knowledge of trending searches, retailers can then be agile with their product discovery strategies. They can take steps to improve and hasten the shopper journey while also using the data to improve product rankings, such as reducing zero results for misspelled queries.
Clickstream behavioral data analysis
When it comes to strategic planning, one of the most reliable sources on shoppers’ interests is shoppers themselves.
That’s why clickstream data, or the trail of your shoppers’ online actions, is such an important indicator of intent. Clickstream data ecompasses what shoppers click on, hover over, spend the most active time viewing, search for, add to cart, remove from cart, purchase, etc.
Product discovery platforms purpose-built for ecommerce use clickstream as a critical signal. This allows fashion retailers to better understand individual shopper behavior, accommodate and anticipate demand, and more easily configure product rankings site-wide.
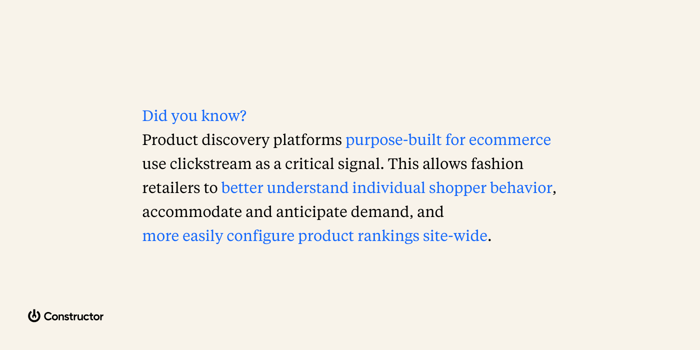
How Generative AI (GenAI) Currently Plays a Role
Thanks to advances in Generative AI (GenAI) technologies, fashion and apparel retailers can more quickly track and predict changes in trends as well. Through this intelligence and automation, they can serve up attractive product recommendations that reflect individual affinities and are optimized for key business KPIs.
Here’s a recent example of how the GenAI-based Attribute Enrichment enabled a major fashion brand to leverage real-time data and capitalize on shopper demand.
When TikTok popularized the term “LumberJane” to refer to baggy fleece and waffle shirts, many apparel merchandisers failed to quickly turn the trend into sales. That is, when shoppers searched “LumberJane” on their sites, they were met with no results. It wasn’t because those types of garments didn’t exist in their inventory, but because the shirts’ product data wasn’t tagged in this way.
Using Attribute Enrichment, the fashion brand was able to enrich product data at the speed of social media. The GenAI-based tool picked up on search and shopping trends and auto-populated “LumberJane” as a searchable product attribute on relevant clothing. In this way, the retailer immediately served up results that aligned to shopper intent and was able to turn demand into sales.
What’s more, results from other retailers using Attribute Enrichment include 97%+ accuracy in product tagging. This is far greater than what’s achieved by humans doing the same tasks, and by also saving precious time.
Combining AI with a Human Touch
Recent advances in AI have enabled retailers to forecast, plan, and meet demand like never before. But does that mean AI is elbowing humans out of the picture?
“Don’t count on it,” says your Magic 8 Ball.
With AI-based tools, fashion merchandisers and ecommerce teams find their menial and highly manual load lightened.
For example, Birkenstock merchandisers reduced manual work by 20% through the use of AI-native solutions. This freed their teams up significantly to focus on more creative and strategic aspects of their jobs.
What’s more, rather than take a “black box” approach, some product discovery engines enable retailers to easily understand and finetune the algorithms that guide product recommendations and search results.
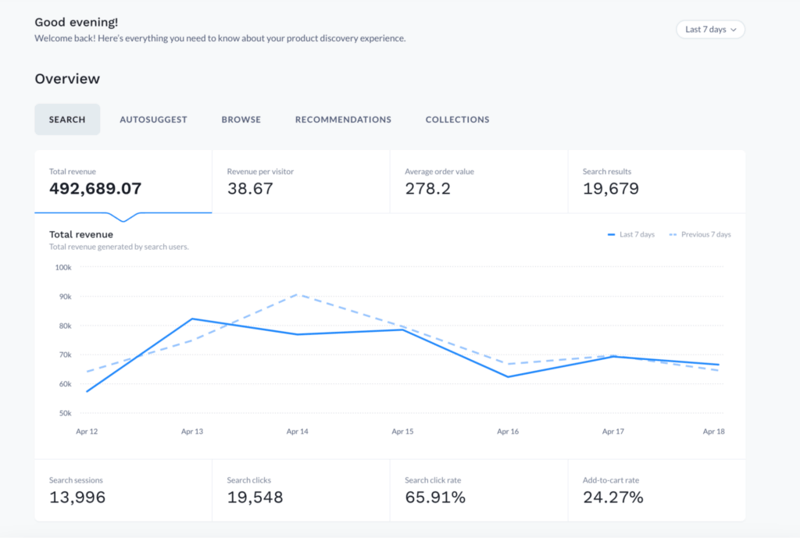
In a recent article in The Guardian, Francesca Muston, VP for fashion at WGSN, explained how human creativity and perspective often greatly complement AI models when it comes to forecasting, putting insights from the models within a wider social context.
“If we’re forecasting, for example, for the beauty industry, that team of people may have worked on formulations of face creams and will have an innate understanding of the product,” she told The Guardian.
Improve Forecasting, Trend-Spotting, and the Bottom Line
Even in its early days, it’s clear that AI-based technologies are having a meaningful impact on fashion retailers’ operations, efficiency, customer experience, and bottom line — especially when it comes to improving forecasting and trend-spotting.
A recent McKinsey survey found that nearly three-quarters (73%) of fashion execs say GenAI will be a priority for their business over the next year. What’s more, in its “Unlocking the Future of Fashion” report, McKinsey predicts that in the next three to five years, GenAI will add between $150 billion and $275 billion to the apparel, fashion, and luxury sectors’ operating profits.
Can your brand effectively harness GenAI for competitive advantage? The Magic 8 Ball says, “Outlook good!”
To learn how to use AI in a way that isn’t just flashy, but also drives measurable value for your customers and business, check out the Constructor ebook, “Data-Driven Merchandising in Fashion & Apparel: Why It’s the Perfect Fit.”